Exploring Algorithms for Revealing Freight Vehicle Tours, Tour-Types, and Tour-Chain-Types from GPS Vehicle Traces and Stop Activity Data
Author(s)
Jing, Peiyu; Ben-Akiva, Moshe E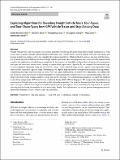
DownloadPublished version (3.582Mb)
Publisher with Creative Commons License
Publisher with Creative Commons License
Creative Commons Attribution
Terms of use
Metadata
Show full item recordAbstract
Freight vehicle tours and tour-chains are essential elements of state-the-art agent-based urban freight simulations as well as key units to analyse freight vehicle demand. GPS traces are typically used to extract vehicle tours and tour-chains and became available in a large scale to, for example, fleet management firms. While methods to process this data with the objec-tive of analysing and modelling tour-based freight vehicle operations have been proposed, they were not fully explored with regard to the implication of underlying assumptions. In this context, we test different algorithms of stop-to-tour assignment, tour-type and tour-chain identification, aiming to expose their implications. Specifically, we compare the traditional stop-to-tour assignment algorithm using the location of a “base” as the start/end point of tours, against other algorithms using stop activities or payload capacity usage. Furthermore, we explore high-resolution tour-type/chain identification algorithms, considering stop types and recurrence of visits. For tour-chain identification, we explore two algorithms: one defines the day-level tour-chain-type based on the predominant tour-type identified for the period of 1 day and another defines the tour-chain-type based on the average number of stops per tour by stop type. For a demonstration purpose, we apply the methods to data from a large-scale GPS-based survey conducted during 2017–2019 in Singapore. We compare the algorithms in an assessment of freight vehicle operations day-to-day pattern homogeneity. Our analysis demonstrates that the predictions of tours, tourtypes, and tour-chain-types are highly dependent on the assumptions used, underlining the importance of carefully selecting and disclosing the methods for data processing. Finally, the exploration of day-to-day pattern homogeneity reveals operational differences across vehicle types and industries.
Date issued
2019-11Department
Massachusetts Institute of Technology. Department of Civil and Environmental Engineering; Massachusetts Institute of Technology. Intelligent Transportation Systems LaboratoryJournal
Journal of Big Data Analytics in Transportation
Publisher
Springer Science and Business Media LLC
Citation
Alho, André Romano et al. “Exploring Algorithms for Revealing Freight Vehicle Tours, Tour-Types, and Tour-Chain-Types from GPS Vehicle Traces and Stop Activity Data” Journal of Big Data Analytics in Transportation, vol. 1, no. 2-3, 2019, pp. 175-190 © 2019 The Author(s)
Version: Final published version
ISSN
2523-3564