Nonparametric System identification of Stochastic Switched Linear Systems
Author(s)
Sarkar, Tuhin; Rakhlin, Alexander; Dahleh, Munther A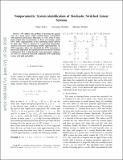
DownloadSubmitted version (200.9Kb)
Open Access Policy
Open Access Policy
Creative Commons Attribution-Noncommercial-Share Alike
Terms of use
Metadata
Show full item recordAbstract
We address the problem of learning the parameters of a mean square stable switched linear systems(SLS) with unknown latent space dimension, or order, from its noisy input-output data. In particular, we focus on learning a good lower order approximation of the underlying model allowed by finite data. This is achieved by constructing Hankel-like matrices from data and obtaining suitable approximations via SVD truncation where the threshold for SVD truncation is purely data dependent. By exploiting tools from theory of model reduction for SLS, we find that the system parameter estimates are close to a balanced truncated realization of the underlying system with high probability.
Date issued
2020-03Department
Massachusetts Institute of Technology. Department of Brain and Cognitive SciencesJournal
Proceedings of the IEEE Conference on Decision and Control
Publisher
Institute of Electrical and Electronics Engineers (IEEE)
Citation
Sarkar, Tuhin et al. “Nonparametric System identification of Stochastic Switched Linear Systems.” Paper in the Proceedings of the IEEE Conference on Decision and Control, 2019-December, 2019 IEEE 58th Conference on Decision and Control (CDC), Nice, France, 11-13 Dec. 2019, IEEE © 2019 The Author(s)
Version: Original manuscript
ISBN
9781728113999
ISSN
0743-1546