Detecting Natural Disasters, Damage, and Incidents in the Wild
Author(s)
Weber, Ethan; Marzo, Nuria; Papadopoulos, Dim P; Biswas, Aritro; Lapedriza Garcia, Agata; Ofli, Ferda; Imran, Muhammad; Torralba, Antonio; ... Show more Show less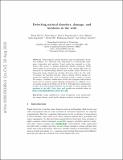
DownloadAccepted version (16.52Mb)
Open Access Policy
Open Access Policy
Creative Commons Attribution-Noncommercial-Share Alike
Terms of use
Metadata
Show full item recordAbstract
Responding to natural disasters, such as earthquakes, floods, and wildfires, is a laborious task performed by on-the-ground emergency responders and analysts. Social media has emerged as a low-latency data source to quickly understand disaster situations. While most studies on social media are limited to text, images offer more information for understanding disaster and incident scenes. However, no large-scale image datasets for incident detection exists. In this work, we present the Incidents Dataset, which contains 446,684 images annotated by humans that cover 43 incidents across a variety of scenes. We employ a baseline classification model that mitigates false-positive errors and we perform image filtering experiments on millions of social media images from Flickr and Twitter. Through these experiments, we show how the Incidents Dataset can be used to detect images with incidents in the wild. Code, data, and models are available online at http://incidentsdataset.csail.mit.edu.
Description
Part of the Lecture Notes in Computer Science book series (LNCS, volume 12364)
Date issued
2020-11Department
Massachusetts Institute of Technology. Department of Electrical Engineering and Computer ScienceJournal
Lecture Notes in Computer Science
Publisher
Springer International Publishing
Citation
Weber, Ethan et al. "Detecting Natural Disasters, Damage, and Incidents in the Wild." ECCV: European Conference on Computer Vision, Lecture Notes in Computer Science, 12364, Springer International Publishing, 2020, 331-350. © 2020 Springer Nature
Version: Author's final manuscript
ISBN
9783030585280
9783030585297
ISSN
0302-9743
1611-3349