An Architecture-Level Energy and Area Estimator for Processing-In-Memory Accelerator Designs
Author(s)
Wu, Yannan Nellie; Sze, Vivienne; Emer, Joel S.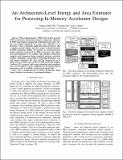
DownloadAccepted version (289.9Kb)
Open Access Policy
Open Access Policy
Creative Commons Attribution-Noncommercial-Share Alike
Terms of use
Metadata
Show full item recordAbstract
Processing-in-memory (PIM) deep neural network (DNN) accelerators, which aim to improve energy/area efficiency of DNN processing by integrating computation into data storage, have gained popularity in recent years. Therefore, it is attractive to have a generally applicable framework that is able to quickly provide insights into the various trade-offs involved in PIM accelerator designs. We present an architecture-level design estimation framework for PIM accelerators that allows easy representations of the designs with provided architecture templates and component design templates, performs analytical runtime simulations, and produces technology-dependent area and energy estimations. We show that the framework can be easily used to evaluate state of the art PIM accelerator designs; it achieves 95% accurate total energy estimations and reproduces exact area breakdowns of the components in the design. Related open-source code is available at http://accelergy.mit.edu/.
Date issued
2020-10Department
Massachusetts Institute of Technology. Department of Electrical Engineering and Computer Science; Massachusetts Institute of Technology. Microsystems Technology LaboratoriesJournal
2020 IEEE International Symposium on Performance Analysis of Systems and Software
Publisher
Institute of Electrical and Electronics Engineers (IEEE)
Citation
Wu, Yannan Nellie et al. "An Architecture-Level Energy and Area Estimator for Processing-In-Memory Accelerator Designs." 2020 IEEE International Symposium on Performance Analysis of Systems and Software. August, 2020, Boston, MA, Institute of Electrical and Electronics Engineers, October 2020. © 2020 IEEE
Version: Author's final manuscript
ISBN
9781728147987