Deep neural network for pixel-level electromagnetic particle identification in the MicroBooNE liquid argon time projection chamber
Author(s)
MicroBooNE Collaboration; Ashkenazi, Adi; Carr, Rachel; Collin, G. H.; Conrad, Janet Marie; Diaz, Alejandro; Hen, Or; Hourlier, Adrien C.; Moon, Joongho; Papadopoulou, Afroditi; Yates, Lauren Elizabeth; ... Show more Show less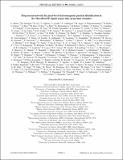
DownloadPublished version (6.161Mb)
Publisher Policy
Publisher Policy
Article is made available in accordance with the publisher's policy and may be subject to US copyright law. Please refer to the publisher's site for terms of use.
Terms of use
Metadata
Show full item recordAbstract
We have developed a convolutional neural network that can make a pixel-level prediction of objects in image data recorded by a liquid argon time projection chamber (LArTPC) for the first time. We describe the network design, training techniques, and software tools developed to train this network. The goal of this work is to develop a complete deep neural network based data reconstruction chain for the MicroBooNE detector. We show the first demonstration of a network's validity on real LArTPC data using MicroBooNE collection plane images. The demonstration is performed for stopping muon and a νμ charged-current neutral pion data samples.
Date issued
2019Department
Massachusetts Institute of Technology. Laboratory for Nuclear Science; Massachusetts Institute of Technology. Department of Physics; Massachusetts Institute of Technology. Department of Nuclear Science and Engineering; Massachusetts Institute of Technology. Institute for Soldier NanotechnologiesJournal
Physical Review D
Publisher
American Physical Society (APS)