Notice
This is not the latest version of this item. The latest version can be found at:https://dspace.mit.edu/handle/1721.1/132287.2
VizNet: Towards A Large-Scale Visualization Learning and Benchmarking Repository
Author(s)
Hu, Kevin; Demiralp, Çağatay; Gaikwad, Snehalkumar 'Neil' S; Hulsebos, Madelon; Bakker, Michiel A; Zgraggen, Emanuel; Hidalgo, César; Kraska, Tim; Li, Guoliang; Satyanarayan, Arvind; ... Show more Show less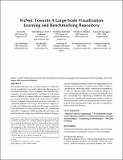
DownloadAccepted version (6.963Mb)
Open Access Policy
Open Access Policy
Creative Commons Attribution-Noncommercial-Share Alike
Terms of use
Metadata
Show full item recordAbstract
© 2019 Copyright held by the owner/author(s). Researchers currently rely on ad hoc datasets to train automated visualization tools and evaluate the efectiveness of visualization designs. These exemplars often lack the characteristics of real-world datasets, and their one-of nature makes it difcult to compare diferent techniques. In this paper, we present VizNet: a large-scale corpus of over 31 million datasets compiled from open data repositories and online visualization galleries. On average, these datasets comprise 17 records over 3 dimensions and across the corpus, we fnd 51% of the dimensions record categorical data, 44% quantitative, and only 5% temporal. VizNet provides the necessary common baseline for comparing visualization design techniques, and developing benchmark models and algorithms for automating visual analysis. To demonstrate VizNet’s utility as a platform for conducting online crowdsourced experiments at scale, we replicate a prior study assessing the infuence of user task and data distribution on visual encoding efectiveness, and extend it by considering an additional task: outlier detection. To contend with running such studies at scale, we demonstrate how a metric of perceptual efectiveness can be learned from experimental results, and show its predictive power across test datasets.
Journal
Conference on Human Factors in Computing Systems - Proceedings
Publisher
Association for Computing Machinery (ACM)