MIRIS: Fast Object Track Queries in Video
Author(s)
Bastani, Favyen; He, Songtao; Balasingam, Arjun; Gopalakrishnan, Karthik; Alizadeh Attar, Mohammadreza; Balakrishnan, Hari; Cafarella, Michael J; Kraska, Tim; Madden, Samuel R; ... Show more Show less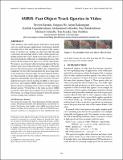
Download3318464.3389692.pdf (7.215Mb)
Publisher Policy
Publisher Policy
Article is made available in accordance with the publisher's policy and may be subject to US copyright law. Please refer to the publisher's site for terms of use.
Terms of use
Metadata
Show full item recordAbstract
© 2020 Association for Computing Machinery. Video databases that enable queries with object-track predicates are useful in many applications. Such queries include selecting objects that move from one region of the camera frame to another (e.g., finding cars that turn right through a junction) and selecting objects with certain speeds (e.g., finding animals that stop to drink water from a lake). Processing such predicates efficiently is challenging because they involve the movement of an object over several video frames. We propose a novel query-driven tracking approach that integrates query processing with object tracking to efficiently process object track queries and address the computational complexity of object detection methods. By processing video at low framerates when possible, but increasing the framerate when needed to ensure high-accuracy on a query, our approach substantially speeds up query execution. We have implemented query-driven tracking in MIRIS, a video query processor, and compare MIRIS against four baselines on a diverse dataset consisting of five sources of video and nine distinct queries. We find that, at the same accuracy, MIRIS accelerates video query processing by 9x on average over the IOU tracker, an overlap-based tracking-by-detection method used in existing video database systems.
Date issued
2020Department
Massachusetts Institute of Technology. Department of Electrical Engineering and Computer Science; Massachusetts Institute of Technology. Computer Science and Artificial Intelligence LaboratoryJournal
Proceedings of the ACM SIGMOD International Conference on Management of Data
Publisher
ACM