Adversarially robust optimization with Gaussian processes
Author(s)
Bogunovic, Ilija; Jegelka, Stefanie Sabrina; Scarlett, Jonathan; Cevher, Volkan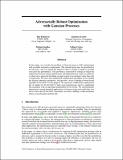
DownloadPublished version (3.120Mb)
Publisher Policy
Publisher Policy
Article is made available in accordance with the publisher's policy and may be subject to US copyright law. Please refer to the publisher's site for terms of use.
Terms of use
Metadata
Show full item recordAbstract
© 2018 Curran Associates Inc.All rights reserved. In this paper, we consider the problem of Gaussian process (GP) optimization with an added robustness requirement: The returned point may be perturbed by an adversary, and we require the function value to remain as high as possible even after this perturbation. This problem is motivated by settings in which the underlying functions during optimization and implementation stages are different, or when one is interested in finding an entire region of good inputs rather than only a single point. We show that standard GP optimization algorithms do not exhibit the desired robustness properties, and provide a novel confidence-bound based algorithm STABLEOPT for this purpose. We rigorously establish the required number of samples for STABLEOPT to find a near-optimal point, and we complement this guarantee with an algorithm-independent lower bound. We experimentally demonstrate several potential applications of interest using real-world data sets, and we show that STABLEOPT consistently succeeds in finding a stable maximizer where several baseline methods fail.
Date issued
2018Department
Massachusetts Institute of Technology. Computer Science and Artificial Intelligence Laboratory; Massachusetts Institute of Technology. Department of Electrical Engineering and Computer ScienceJournal
Advances in Neural Information Processing Systems