CryoDRGN: reconstruction of heterogeneous cryo-EM structures using neural networks
Author(s)
Zhong, Ellen D; Bepler, Tristan; Berger, Bonnie; Davis, Joseph H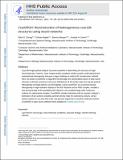
DownloadAccepted version (5.639Mb)
Open Access Policy
Open Access Policy
Creative Commons Attribution-Noncommercial-Share Alike
Terms of use
Metadata
Show full item recordAbstract
© 2021, The Author(s), under exclusive licence to Springer Nature America, Inc. Cryo-electron microscopy (cryo-EM) single-particle analysis has proven powerful in determining the structures of rigid macromolecules. However, many imaged protein complexes exhibit conformational and compositional heterogeneity that poses a major challenge to existing three-dimensional reconstruction methods. Here, we present cryoDRGN, an algorithm that leverages the representation power of deep neural networks to directly reconstruct continuous distributions of 3D density maps and map per-particle heterogeneity of single-particle cryo-EM datasets. Using cryoDRGN, we uncovered residual heterogeneity in high-resolution datasets of the 80S ribosome and the RAG complex, revealed a new structural state of the assembling 50S ribosome, and visualized large-scale continuous motions of a spliceosome complex. CryoDRGN contains interactive tools to visualize a dataset’s distribution of per-particle variability, generate density maps for exploratory analysis, extract particle subsets for use with other tools and generate trajectories to visualize molecular motions. CryoDRGN is open-source software freely available at http://cryodrgn.csail.mit.edu.
Date issued
2021Department
Massachusetts Institute of Technology. Computational and Systems Biology Program; Massachusetts Institute of Technology. Computer Science and Artificial Intelligence Laboratory; Massachusetts Institute of Technology. Department of Mathematics; Massachusetts Institute of Technology. Department of BiologyJournal
Nature Methods
Publisher
Springer Science and Business Media LLC