Forecasts of Hurricanes Using Large-Ensemble Outputs
Author(s)
Lin, Jonathan; Emanuel, Kerry; Vigh, Jonathan L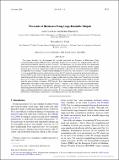
DownloadPublished version (6.986Mb)
Publisher Policy
Publisher Policy
Article is made available in accordance with the publisher's policy and may be subject to US copyright law. Please refer to the publisher's site for terms of use.
Terms of use
Metadata
Show full item recordAbstract
© 2020 American Meteorological Society. This paper describes the development of a model framework for Forecasts of Hurricanes Using Large-Ensemble Outputs (FHLO). FHLO quantifies the forecast uncertainty of a tropical cyclone (TC) by generating probabilistic forecasts of track, intensity, and wind speed that incorporate the state-dependent uncertainty in the large-scale field. The main goal is to provide useful probabilistic forecasts of wind at fixed points in space, but these require large ensembles [O(1000)] to flesh out the tails of the distributions. FHLO accomplishes this by using a computationally inexpensive framework, which consists of three components: 1) a track model that generates synthetic tracks from the TC tracks of an ensemble numerical weather prediction (NWP) model, 2) an intensity model that predicts the intensity along each synthetic track, and 3) a TC wind field model that estimates the time-varying two-dimensional surface wind field. The intensity and wind field of a TC evolve as though the TC were embedded in a time-evolving environmental field, which is derived from the forecast fields of ensemble NWP models. Each component of the framework is evaluated using 1000-member ensembles and four years (2015–18) of TC forecasts in the Atlantic and eastern Pacific basins. We show that the synthetic track algorithm generates tracks that are statistically similar to those of the underlying global ensemble models. We show that FHLO produces competitive intensity forecasts, especially when considering probabilistic verification statistics. We also demonstrate the reliability and accuracy of the probabilistic wind forecasts. Limitations of the model framework are also discussed.
Date issued
2020-10Department
Massachusetts Institute of Technology. Department of Earth, Atmospheric, and Planetary Sciences; Lorenz Center (Massachusetts Institute of Technology)Journal
Weather and Forecasting
Publisher
American Meteorological Society
ISSN
1520-0434
0882-8156