Machine learning optimization of peptides for presentation by class II MHCs
Author(s)
Dai, Zheng; Huisman, Brooke D; Zeng, Haoyang; Carter, Brandon; Jain, Siddhartha; Birnbaum, Michael E; Gifford, David K; ... Show more Show less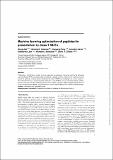
DownloadPublished version (1.716Mb)
Publisher with Creative Commons License
Publisher with Creative Commons License
Creative Commons Attribution
Terms of use
Metadata
Show full item recordAbstract
<jats:title>Abstract</jats:title>
<jats:sec>
<jats:title>Summary</jats:title>
<jats:p>T cells play a critical role in cellular immune responses to pathogens and cancer and can be activated and expanded by Major Histocompatibility Complex (MHC)-presented antigens contained in peptide vaccines. We present a machine learning method to optimize the presentation of peptides by class II MHCs by modifying their anchor residues. Our method first learns a model of peptide affinity for a class II MHC using an ensemble of deep residual networks, and then uses the model to propose anchor residue changes to improve peptide affinity. We use a high throughput yeast display assay to show that anchor residue optimization improves peptide binding.</jats:p>
</jats:sec>
<jats:sec>
<jats:title>Supplementary information</jats:title>
<jats:p>Supplementary data are available at Bioinformatics online.</jats:p>
</jats:sec>
Date issued
2021Department
Massachusetts Institute of Technology. Computer Science and Artificial Intelligence Laboratory; Massachusetts Institute of Technology. Department of Electrical Engineering and Computer Science; Massachusetts Institute of Technology. Department of Biological EngineeringJournal
Bioinformatics
Publisher
Oxford University Press (OUP)