Machine learning for deep elastic strain engineering of semiconductor electronic band structure and effective mass
Author(s)
Tsymbalov, Evgenii; Shi, Zhe; Dao, Ming; Suresh, Subra; Li, Ju; Shapeev, Alexander; ... Show more Show less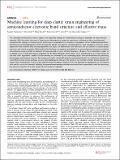
DownloadPublished version (1.994Mb)
Publisher with Creative Commons License
Publisher with Creative Commons License
Creative Commons Attribution
Terms of use
Metadata
Show full item recordAbstract
<jats:title>Abstract</jats:title><jats:p>The controlled introduction of elastic strains is an appealing strategy for modulating the physical properties of semiconductor materials. With the recent discovery of large elastic deformation in nanoscale specimens as diverse as silicon and diamond, employing this strategy to improve device performance necessitates first-principles computations of the fundamental electronic band structure and target figures-of-merit, through the design of an optimal straining pathway. Such simulations, however, call for approaches that combine deep learning algorithms and physics of deformation with band structure calculations to custom-design electronic and optical properties. Motivated by this challenge, we present here details of a machine learning framework involving convolutional neural networks to represent the topology and curvature of band structures in <jats:bold>k</jats:bold>-space. These calculations enable us to identify ways in which the physical properties can be altered through “deep” elastic strain engineering up to a large fraction of the ideal strain. Algorithms capable of active learning and informed by the underlying physics were presented here for predicting the bandgap and the band structure. By training a surrogate model with ab initio computational data, our method can identify the most efficient strain energy pathway to realize physical property changes. The power of this method is further demonstrated with results from the prediction of strain states that influence the effective electron mass. We illustrate the applications of the method with specific results for diamonds, although the general deep learning technique presented here is potentially useful for optimizing the physical properties of a wide variety of semiconductor materials.</jats:p>
Date issued
2021Department
Massachusetts Institute of Technology. Department of Materials Science and Engineering; Massachusetts Institute of Technology. Department of Nuclear Science and EngineeringJournal
npj Computational Materials
Publisher
Springer Science and Business Media LLC