Locality Preserving Discriminative Canonical Variate Analysis for Fault Diagnosis
Author(s)
Lu, Qiugang; Jiang, Benben; Gopaluni, R Bhushan; Loewen, Philip D; Braatz, Richard D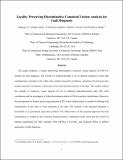
DownloadAccepted version (2.158Mb)
Publisher with Creative Commons License
Publisher with Creative Commons License
Creative Commons Attribution
Terms of use
Metadata
Show full item recordAbstract
© 2018 Elsevier Ltd This paper proposes a locality preserving discriminative canonical variate analysis (LP-DCVA) scheme for fault diagnosis. The LP-DCVA method provides a set of optimal projection vectors that simultaneously maximizes the within-class mutual canonical correlations, minimizes the between-class mutual canonical correlations, and preserves the local structures present in the data. This method inherits the strength of canonical variate analysis (CVA) in handling high-dimensional data with serial correlations and the advantages of Fisher discriminant analysis (FDA) in pattern classification. Moreover, the incorporation of locality preserving projection (LPP) in this method makes it suitable for dealing with nonlinearities in the form of local manifolds in the data. The solution to the proposed approach is formulated as a generalized eigenvalue problem. The effectiveness of the proposed approach for fault classification is verified by the Tennessee Eastman process. Simulation results show that the LP-DCVA method outperforms the FDA, dynamic FDA (DFDA), CVA-FDA, and localized DFDA (L-DFDA) approaches in fault diagnosis.
Date issued
2018Department
Massachusetts Institute of Technology. Department of Chemical EngineeringJournal
Computers and Chemical Engineering
Publisher
Elsevier BV