Notice
This is not the latest version of this item. The latest version can be found at:https://dspace.mit.edu/handle/1721.1/136301.2
Complexity control by gradient descent in deep networks
Author(s)
Poggio, Tomaso; Liao, Qianli; Banburski, Andrzej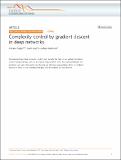
DownloadPublished version (431.6Kb)
Publisher with Creative Commons License
Publisher with Creative Commons License
Creative Commons Attribution
Terms of use
Metadata
Show full item recordAbstract
© 2020, The Author(s). Overparametrized deep networks predict well, despite the lack of an explicit complexity control during training, such as an explicit regularization term. For exponential-type loss functions, we solve this puzzle by showing an effective regularization effect of gradient descent in terms of the normalized weights that are relevant for classification.
Date issued
2020Journal
Nature Communications
Publisher
Springer Science and Business Media LLC