Combined burden and functional impact tests for cancer driver discovery using DriverPower
Author(s)
Shuai, Shimin; Gallinger, Steven; Stein, Lincoln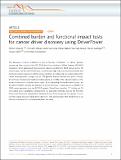
DownloadPublished version (676.4Kb)
Publisher with Creative Commons License
Publisher with Creative Commons License
Creative Commons Attribution
Terms of use
Metadata
Show full item recordAbstract
<jats:title>Abstract</jats:title><jats:p>The discovery of driver mutations is one of the key motivations for cancer genome sequencing. <jats:italic>Here</jats:italic>, <jats:italic>as part of the ICGC/TCGA Pan-Cancer Analysis of Whole Genomes (PCAWG) Consortium</jats:italic>, which aggregated whole genome sequencing data from 2658 cancers across 38 tumour types, we describe DriverPower, a software package that uses mutational burden and functional impact evidence to identify driver mutations in coding and non-coding sites within cancer whole genomes. Using a total of 1373 genomic features derived from public sources, DriverPower’s background mutation model explains up to 93% of the regional variance in the mutation rate across multiple tumour types. By incorporating functional impact scores, we are able to further increase the accuracy of driver discovery. Testing across a collection of 2583 cancer genomes from the PCAWG project, DriverPower identifies 217 coding and 95 non-coding driver candidates. Comparing to six published methods used by the PCAWG Drivers and Functional Interpretation Working Group, DriverPower has the highest F1 score for both coding and non-coding driver discovery. This demonstrates that DriverPower is an effective framework for computational driver discovery.</jats:p>
Date issued
2020-02Department
Massachusetts Institute of Technology. Computer Science and Artificial Intelligence LaboratoryJournal
Nature Communications
Publisher
Springer Science and Business Media LLC
ISSN
2041-1723