Enhancing searches for resonances with machine learning and moment decomposition
Author(s)
Kitouni, Ouail; Nachman, Benjamin; Weisser, Constantin; Williams, Mike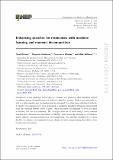
Download13130_2021_Article_15327.pdf (610.1Kb)
Publisher with Creative Commons License
Publisher with Creative Commons License
Creative Commons Attribution
Terms of use
Metadata
Show full item recordAbstract
Abstract
A key challenge in searches for resonant new physics is that classifiers trained to enhance potential signals must not induce localized structures. Such structures could result in a false signal when the background is estimated from data using sideband methods. A variety of techniques have been developed to construct classifiers which are independent from the resonant feature (often a mass). Such strategies are sufficient to avoid localized structures, but are not necessary. We develop a new set of tools using a novel moment loss function (Moment Decomposition or MoDe) which relax the assumption of independence without creating structures in the background. By allowing classifiers to be more flexible, we enhance the sensitivity to new physics without compromising the fidelity of the background estimation.
Date issued
2021-04-08Department
Massachusetts Institute of Technology. Laboratory for Nuclear Science; Massachusetts Institute of Technology. Institute for Data, Systems, and Society; Statistics and Data Science Center (Massachusetts Institute of Technology)Publisher
Springer Berlin Heidelberg
Citation
Journal of High Energy Physics. 2021 Apr 08;2021(4):70
Version: Final published version