CoCoA-diff: counterfactual inference for single-cell gene expression analysis
Author(s)
Park, Yongjin P.; Kellis, Manolis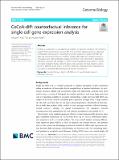
Download13059_2021_Article_2438.pdf (3.030Mb)
Publisher with Creative Commons License
Publisher with Creative Commons License
Creative Commons Attribution
Terms of use
Metadata
Show full item recordAbstract
Abstract
Finding a causal gene is a fundamental problem in genomic medicine. We present a causal inference framework, CoCoA-diff, that prioritizes disease genes by adjusting confounders without prior knowledge of control variables in single-cell RNA-seq data. We demonstrate that our method substantially improves statistical power in simulations and real-world data analysis of 70k brain cells collected for dissecting Alzheimer’s disease. We identify 215 differentially regulated causal genes in various cell types, including highly relevant genes with a proper cell type context. Genes found in different types enrich distinctive pathways, implicating the importance of cell types in understanding multifaceted disease mechanisms.
Date issued
2021-08Department
Massachusetts Institute of Technology. Computer Science and Artificial Intelligence LaboratoryJournal
Genome Biology
Publisher
Springer Science and Business Media LLC
Citation
Genome Biology. 2021 Aug 17;22(1):228
Version: Final published version
ISSN
1474-760X