Quantitative tumor heterogeneity MRI profiling improves machine learning–based prognostication in patients with metastatic colon cancer
Author(s)
Daye, Dania; Tabari, Azadeh; Kim, Hyunji; Chang, Ken; Kamran, Sophia C.; Hong, Theodore S.; Kalpathy-Cramer, Jayashree; Gee, Michael S.; ... Show more Show less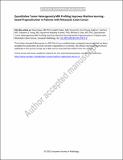
Download330_2020_7673_ReferencePDF.pdf (1.706Mb)
Publisher Policy
Publisher Policy
Article is made available in accordance with the publisher's policy and may be subject to US copyright law. Please refer to the publisher's site for terms of use.
Terms of use
Metadata
Show full item recordAbstract
Abstract
Objectives
Intra-tumor heterogeneity has been previously shown to be an independent predictor of patient survival. The goal of this study is to assess the role of quantitative MRI-based measures of intra-tumor heterogeneity as predictors of survival in patients with metastatic colorectal cancer.
Methods
In this IRB-approved retrospective study, we identified 55 patients with stage 4 colon cancer with known hepatic metastasis on MRI. Ninety-four metastatic hepatic lesions were identified on post-contrast images and manually volumetrically segmented. A heterogeneity phenotype vector was extracted from each lesion. Univariate regression analysis was used to assess the contribution of 110 extracted features to survival prediction. A random forest–based machine learning technique was applied to the feature vector and to the standard prognostic clinical and pathologic variables. The dataset was divided into a training and test set at a ratio of 4:1. ROC analysis and confusion matrix analysis were used to assess classification performance.
Results
Mean survival time was 39 ± 3.9 months for the study population. A total of 22 texture features were associated with patient survival (p < 0.05). The trained random forest machine learning model that included standard clinical and pathological prognostic variables resulted in an area under the ROC curve of 0.83. A model that adds imaging-based heterogeneity features to the clinical and pathological variables resulted in improved model performance for survival prediction with an AUC of 0.94.
Conclusions
MRI-based texture features are associated with patient outcomes and improve the performance of standard clinical and pathological variables for predicting patient survival in metastatic colorectal cancer.
Key Points
• MRI-based tumor heterogeneity texture features are associated with patient survival outcomes.
• MRI-based tumor texture features complement standard clinical and pathological variables for prognosis prediction in metastatic colorectal cancer.
• Agglomerative hierarchical clustering shows that patient survival outcomes are associated with different MRI tumor profiles.
Date issued
2021-01Department
Massachusetts Institute of Technology. Computer Science and Artificial Intelligence LaboratoryJournal
European Radiology
Publisher
Springer Science and Business Media LLC
ISSN
0938-7994
1432-1084