Improved bounds on Gaussian MAC and sparse regression via Gaussian inequalities
Author(s)
Zadik, Ilias; Polyanskiy, Yury; Thrampoulidis, Christos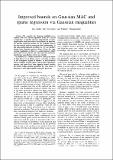
DownloadAccepted version (295.2Kb)
Open Access Policy
Open Access Policy
Creative Commons Attribution-Noncommercial-Share Alike
Terms of use
Metadata
Show full item recordAbstract
© 2019 IEEE. We consider the Gaussian multiple-access channel with two critical departures from the classical asymptotics: a) number of users proportional to block-length and b) each user sends a fixed number of data bits. We provide improved bounds on the tradeoff between the user density and the energy-per-bit. Interestingly, in this information-theoretic problem we rely on Gordon's lemma from Gaussian process theory. From the engineering standpoint, we discover a surprising new effect: good coded-access schemes can achieve perfect multi-user interference cancellation at low user density.In addition, by a similar method we analyze the limits of false-discovery in binary sparse regression problem in the asymptotic regime of number of measurements going to infinity at fixed ratios with problem dimension, sparsity and noise level. Our rigorous bound matches the formal replica-method prediction for some range of parameters with imperceptible numerical precision.
Date issued
2019-09Department
Massachusetts Institute of Technology. Department of Electrical Engineering and Computer ScienceJournal
IEEE International Symposium on Information Theory - Proceedings
Publisher
Institute of Electrical and Electronics Engineers (IEEE)
Citation
Zadik, Ilias, Polyanskiy, Yury and Thrampoulidis, Christos. 2019. "Improved bounds on Gaussian MAC and sparse regression via Gaussian inequalities." IEEE International Symposium on Information Theory - Proceedings, 2019-July.
Version: Author's final manuscript