Notice
This is not the latest version of this item. The latest version can be found at:https://dspace.mit.edu/handle/1721.1/137178.2
Meta-Sim: Learning to Generate Synthetic Datasets
Author(s)
Unknown author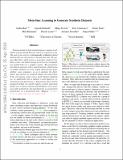
DownloadSubmitted version (8.543Mb)
Open Access Policy
Open Access Policy
Creative Commons Attribution-Noncommercial-Share Alike
Terms of use
Metadata
Show full item recordAbstract
© 2019 IEEE. Training models to high-end performance requires availability of large labeled datasets, which are expensive to get. The goal of our work is to automatically synthesize labeled datasets that are relevant for a downstream task. We propose Meta-Sim, which learns a generative model of synthetic scenes, and obtain images as well as its corresponding ground-truth via a graphics engine. We parametrize our dataset generator with a neural network, which learns to modify attributes of scene graphs obtained from probabilistic scene grammars, so as to minimize the distribution gap between its rendered outputs and target data. If the real dataset comes with a small labeled validation set, we additionally aim to optimize a meta-objective, i.e. downstream task performance. Experiments show that the proposed method can greatly improve content generation quality over a human-engineered probabilistic scene grammar, both qualitatively and quantitatively as measured by performance on a downstream task.
Date issued
2020-10Journal
Proceedings of the IEEE International Conference on Computer Vision
Publisher
IEEE
Citation
2020. "Meta-Sim: Learning to Generate Synthetic Datasets." Proceedings of the IEEE International Conference on Computer Vision, 2019-October.
Version: Original manuscript