Size of interventional Markov equivalence classes in random DAG models
Author(s)
Katz, Dmitriy; Shanmugam, Karthikeyan; Uhler, Caroline; Squires, Chandler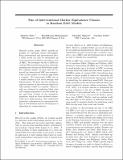
DownloadPublished version (715.4Kb)
Publisher Policy
Publisher Policy
Article is made available in accordance with the publisher's policy and may be subject to US copyright law. Please refer to the publisher's site for terms of use.
Terms of use
Metadata
Show full item recordAbstract
© 2019 by the author(s). Directed acyclic graph (DAG) models are popular for capturing causal relationships. From observational and interventional data, a DAG model can only be determined up to its interventional Markov equivalence class (I-MEC). We investigate the size of MECs for random DAG models generated by uniformly sampling and ordering an Erdős-Rényi graph. For constant density, we show that the expected log observational MEC size asymptotically (in the number of vertices) approaches a constant. We characterize I-MEC size in a similar fashion in the above settings with high precision. We show that the asymptotic expected number of interventions required to fully identify a DAG is a constant. These results are obtained by exploiting Meek rules and coupling arguments to provide sharp upper and lower bounds on the asymptotic quantities, which are then calculated numerically up to high precision. Our results have important consequences for experimental design of interventions and the development of algorithms for causal inference.
Date issued
2019Department
MIT-IBM Watson AI Lab; Massachusetts Institute of Technology. Computer Science and Artificial Intelligence LaboratoryJournal
AISTATS 2019 - 22nd International Conference on Artificial Intelligence and Statistics
Citation
Uhler, Caroline and Squires, Chandler. 2019. "Size of interventional Markov equivalence classes in random DAG models." AISTATS 2019 - 22nd International Conference on Artificial Intelligence and Statistics, 89.
Version: Final published version