Alleviating label switching with optimal transport
Author(s)
Monteiller, Pierre; Claici, Sebastian; Chien, Edward; Mirzazadeh, Farzaneh; Solomon, Justin; Yurochkin, Mikhail; ... Show more Show less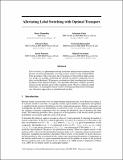
DownloadPublished version (3.683Mb)
Publisher Policy
Publisher Policy
Article is made available in accordance with the publisher's policy and may be subject to US copyright law. Please refer to the publisher's site for terms of use.
Terms of use
Metadata
Show full item recordAbstract
© 2019 Neural information processing systems foundation. All rights reserved. Label switching is a phenomenon arising in mixture model posterior inference that prevents one from meaningfully assessing posterior statistics using standard Monte Carlo procedures. This issue arises due to invariance of the posterior under actions of a group; for example, permuting the ordering of mixture components has no effect on the likelihood. We propose a resolution to label switching that leverages machinery from optimal transport. Our algorithm efficiently computes posterior statistics in the quotient space of the symmetry group. We give conditions under which there is a meaningful solution to label switching and demonstrate advantages over alternative approaches on simulated and real data.
Date issued
2019-12Department
Massachusetts Institute of Technology. Computer Science and Artificial Intelligence Laboratory; MIT-IBM Watson AI LabJournal
Advances in Neural Information Processing Systems
Citation
2019. "Alleviating label switching with optimal transport." Advances in Neural Information Processing Systems, 32.
Version: Final published version