Hierarchical optimal transport for document representation
Author(s)
Yurochkin, Mikhail; Claici, Sebastian; Chien, Edward; Mirzazadeh, Farzaneh; Solomon, Justin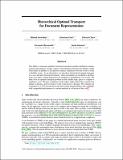
DownloadPublished version (2.297Mb)
Publisher Policy
Publisher Policy
Article is made available in accordance with the publisher's policy and may be subject to US copyright law. Please refer to the publisher's site for terms of use.
Terms of use
Metadata
Show full item recordAbstract
© 2019 Neural information processing systems foundation. All rights reserved. The ability to measure similarity between documents enables intelligent summarization and analysis of large corpora. Past distances between documents suffer from either an inability to incorporate semantic similarities between words or from scalability issues. As an alternative, we introduce hierarchical optimal transport as a meta-distance between documents, where documents are modeled as distributions over topics, which themselves are modeled as distributions over words. We then solve an optimal transport problem on the smaller topic space to compute a similarity score. We give conditions on the topics under which this construction defines a distance, and we relate it to the word mover's distance. We evaluate our technique for k-NN classification and show better interpretability and scalability with comparable performance to current methods at a fraction of the cost.
Date issued
2019-12Department
Massachusetts Institute of Technology. Computer Science and Artificial Intelligence Laboratory; MIT-IBM Watson AI Lab; Massachusetts Institute of Technology. Department of Electrical Engineering and Computer ScienceJournal
Advances in Neural Information Processing Systems
Citation
2019. "Hierarchical optimal transport for document representation." Advances in Neural Information Processing Systems, 32.
Version: Final published version