PRNet: Self-supervised learning for partial-to-partial registration
Author(s)
Wang, Yue; Solomon, Justin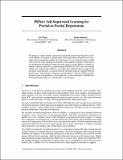
DownloadPublished version (5.370Mb)
Publisher Policy
Publisher Policy
Article is made available in accordance with the publisher's policy and may be subject to US copyright law. Please refer to the publisher's site for terms of use.
Terms of use
Metadata
Show full item recordAbstract
© 2019 Neural information processing systems foundation. All rights reserved. We present a simple, flexible, and general framework titled Partial Registration Network (PRNet), for partial-to-partial point cloud registration. Inspired by recently-proposed learning-based methods for registration, we use deep networks to tackle non-convexity of the alignment and partial correspondence problems. While previous learning-based methods assume the entire shape is visible, PRNet is suitable for partial-to-partial registration, outperforming PointNetLK, DCP, and non-learning methods on synthetic data. PRNet is self-supervised, jointly learning an appropriate geometric representation, a keypoint detector that finds points in common between partial views, and keypoint-to-keypoint correspondences. We show PRNet predicts keypoints and correspondences consistently across views and objects. Furthermore, the learned representation is transferable to classification.
Date issued
2019-12Department
Massachusetts Institute of Technology. Computer Science and Artificial Intelligence Laboratory; Massachusetts Institute of Technology. Department of Electrical Engineering and Computer ScienceJournal
Advances in Neural Information Processing Systems
Citation
2019. "PRNet: Self-supervised learning for partial-to-partial registration." Advances in Neural Information Processing Systems, 32.
Version: Final published version