Multi-Source Domain Adaptation with Mixture of Experts
Author(s)
Guo, Jiang; Shah, Darsh; Barzilay, Regina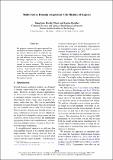
DownloadPublished version (882.5Kb)
Publisher with Creative Commons License
Publisher with Creative Commons License
Creative Commons Attribution
Terms of use
Metadata
Show full item recordAbstract
© 2018 Association for Computational Linguistics We propose a mixture-of-experts approach for unsupervised domain adaptation from multiple sources. The key idea is to explicitly capture the relationship between a target example and different source domains. This relationship, expressed by a point-to-set metric, determines how to combine predictors trained on various domains. The metric is learned in an unsupervised fashion using meta-training. Experimental results on sentiment analysis and part-of-speech tagging demonstrate that our approach consistently outperforms multiple baselines and can robustly handle negative transfer.1
Date issued
2018-10Department
Massachusetts Institute of Technology. Computer Science and Artificial Intelligence LaboratoryJournal
Proceedings of the 2018 Conference on Empirical Methods in Natural Language Processing, EMNLP 2018
Publisher
Association for Computational Linguistics (ACL)
Citation
Guo, Jiang, Shah, Darsh and Barzilay, Regina. 2018. "Multi-Source Domain Adaptation with Mixture of Experts." Proceedings of the 2018 Conference on Empirical Methods in Natural Language Processing, EMNLP 2018.
Version: Final published version