A weighted sparse-input neural network technique applied to identify important features for vortex-induced vibration
Author(s)
Ma, Leixin; Resvanis, Themistocles L.; Vandiver, J. Kim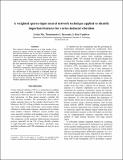
DownloadPublished version (900.9Kb)
Publisher with Creative Commons License
Publisher with Creative Commons License
Creative Commons Attribution
Terms of use
Metadata
Show full item recordAbstract
Copyright © 2020, for this paper by its authors. Flow-induced vibration depends on a large number of parameters or features. On the one hand, the number of candidate physical features may be too big to construct an interpretable and transferrable model. On the other hand, failure to account for key dependence among features may oversimplify the model. Feature selection is found to be able to reduce the dimension of the physical problem by identifying the most important features for a certain prediction task. In this paper, a weighted sparse-input neural network (WSPINN) is proposed, where the prior physical knowledge is leveraged to constrain the neural network optimization. The effectiveness of this approach is evaluated when applied to the vortex-induced vibration of a long flexible cylinder with Reynolds number from 104 to 105. The important physical features affecting the flexible cylinders’ crossflow vibration amplitude are identified.
Date issued
2020Department
Massachusetts Institute of Technology. Department of Mechanical EngineeringJournal
CEUR Workshop Proceedings
Citation
Ma, Leixin, Resvanis, Themistocles L. and Vandiver, J. Kim. 2020. "A weighted sparse-input neural network technique applied to identify important features for vortex-induced vibration." CEUR Workshop Proceedings, 2587.
Version: Final published version