Fast recovery from a union of subspaces
Author(s)
Hegde, Chinmay; Indyk, Piotr; Schmidt, Ludwig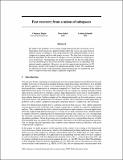
DownloadPublished version (371.8Kb)
Publisher Policy
Publisher Policy
Article is made available in accordance with the publisher's policy and may be subject to US copyright law. Please refer to the publisher's site for terms of use.
Terms of use
Metadata
Show full item recordAbstract
© 2016 NIPS Foundation - All Rights Reserved. We address the problem of recovering a high-dimensional but structured vector from linear observations in a general setting where the vector can come from an arbitrary union of subspaces. This setup includes well-studied problems such as compressive sensing and low-rank matrix recovery. We show how to design more efficient algorithms for the union-of-subspace recovery problem by using approximate projections. Instantiating our general framework for the low-rank matrix recovery problem gives the fastest provable running time for an algorithm with optimal sample complexity. Moreover, we give fast approximate projections for 2D histograms, another well-studied low-dimensional model of data. We complement our theoretical results with experiments demonstrating that our framework also leads to improved time and sample complexity empirically.
Date issued
2016-12Department
Massachusetts Institute of Technology. Department of Electrical Engineering and Computer Science; Massachusetts Institute of Technology. Computer Science and Artificial Intelligence LaboratoryJournal
NIPS'16: Proceedings of the 30th International Conference on Neural Information Processing Systems
Publisher
Curran Associates Inc.
Citation
Indyk, Piotr and Schmidt, Ludwig. 2016. "Fast recovery from a union of subspaces."
Version: Final published version
ISBN
978-1-5108-3881-9