HCU400: an Annotated Dataset for Exploring Aural Phenomenology through Causal Uncertainty
Author(s)
Ananthabhotla, Ishwarya; Ramsay, David B.; Paradiso, Joseph A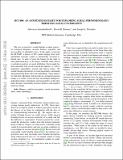
DownloadSubmitted version (2.192Mb)
Open Access Policy
Open Access Policy
Creative Commons Attribution-Noncommercial-Share Alike
Terms of use
Metadata
Show full item recordAbstract
© 2019 IEEE. The way we perceive a sound depends on many aspects- its ecological frequency, acoustic features, typicality, and most notably, its identified source. In this paper, we present the HCU400: a dataset of 402 sounds ranging from easily identifiable everyday sounds to intentionally obscured artificial ones. It aims to lower the barrier for the study of aural phenomenology as the largest available audio dataset to include an analysis of causal attribution. Each sample has been annotated with crowd-sourced descriptions, as well as familiarity, imageability, arousal, and valence ratings. We extend existing calculations of causal uncertainty, automating and generalizing them with word embeddings. Upon analysis we find that individuals will provide less polarized emotion ratings as a sound's source becomes increasingly ambiguous; individual ratings of familiarity and imageability, on the other hand, diverge as uncertainty increases despite a clear negative trend on average.
Date issued
2019-05Department
Massachusetts Institute of Technology. Media LaboratoryPublisher
IEEE
Citation
Ananthabhotla, Ishwarya, Ramsay, David B. and Paradiso, Joseph A. 2019. "HCU400: an Annotated Dataset for Exploring Aural Phenomenology through Causal Uncertainty."
Version: Original manuscript