Weighted message passing and minimum energy flow for heterogeneous stochastic block models with side information
Author(s)
Tony Cai, T; Liang, T; Rakhlin, A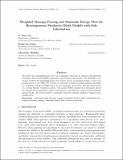
DownloadPublished version (440.9Kb)
Publisher with Creative Commons License
Publisher with Creative Commons License
Creative Commons Attribution
Terms of use
Metadata
Show full item recordAbstract
© 2020 T. Tony Cai, Tengyuan Liang and Alexander Rakhlin. License: CC-BY 4.0, see https://creativecommons.org/licenses/by/4.0/. Attribution requirements are provided at http://jmlr.org/papers/v21/18-573.html. We study the misclassification error for community detection in general heterogeneous stochastic block models (SBM) with noisy or partial label information. We establish a connection between the misclassification rate and the notion of minimum energy on the local neighborhood of the SBM. We develop an optimally weighted message passing algorithm to reconstruct labels for SBM based on the minimum energy flow and the eigenvectors of a certain Markov transition matrix. The general SBM considered in this paper allows for unequal-size communities, degree heterogeneity, and different connection probabilities among blocks. We focus on how to optimally weigh the message passing to improve misclassification.
Date issued
2020-01-01Department
Massachusetts Institute of Technology. Institute for Data, Systems, and SocietyJournal
Journal of Machine Learning Research
Citation
Tony Cai, T, Liang, T and Rakhlin, A. 2020. "Weighted message passing and minimum energy flow for heterogeneous stochastic block models with side information." Journal of Machine Learning Research, 21.
Version: Final published version