Using ℓ1-Relaxation and Integer Programming to Obtain Dual Bounds for Sparse PCA
Author(s)
Dey, Santanu S; Mazumder, Rahul; Wang, Guanyi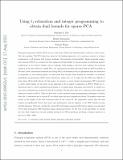
DownloadAccepted version (474.5Kb)
Open Access Policy
Open Access Policy
Creative Commons Attribution-Noncommercial-Share Alike
Terms of use
Metadata
Show full item recordAbstract
<jats:p> Dual Bounds of Sparse Principal Component Analysis </jats:p><jats:p> Sparse principal component analysis (PCA) is a widely used dimensionality reduction tool in machine learning and statistics. Compared with PCA, sparse PCA enhances the interpretability by incorporating a sparsity constraint. However, unlike PCA, conventional heuristics for sparse PCA cannot guarantee the qualities of obtained primal feasible solutions via associated dual bounds in a tractable fashion without underlying statistical assumptions. In “Using L1-Relaxation and Integer Programming to Obtain Dual Bounds for Sparse PCA,” Santanu S. Dey, Rahul Mazumder, and Guanyi Wang present a convex integer programming (IP) framework of sparse PCA to derive dual bounds. They show the worst-case results on the quality of the dual bounds provided by the convex IP. Moreover, the authors empirically illustrate that the proposed convex IP framework outperforms existing sparse PCA methods of finding dual bounds. </jats:p>
Date issued
2021Department
Massachusetts Institute of Technology. Operations Research CenterJournal
Operations Research
Publisher
Institute for Operations Research and the Management Sciences (INFORMS)
Citation
Dey, Santanu S, Mazumder, Rahul and Wang, Guanyi. 2021. "Using ℓ1-Relaxation and Integer Programming to Obtain Dual Bounds for Sparse PCA." Operations Research, 70 (3).
Version: Author's final manuscript