Multi-animal pose estimation, identification and tracking with DeepLabCut
Author(s)
Lauer, Jessy; Zhou, Mu; Ye, Shaokai; Menegas, William; Schneider, Steffen; Nath, Tanmay; Rahman, Mohammed Mostafizur; Di Santo, Valentina; Soberanes, Daniel; Feng, Guoping; Murthy, Venkatesh N; Lauder, George; Dulac, Catherine; Mathis, Mackenzie Weygandt; Mathis, Alexander; ... Show more Show less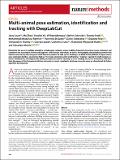
DownloadPublished version (11.60Mb)
Publisher with Creative Commons License
Publisher with Creative Commons License
Creative Commons Attribution
Terms of use
Metadata
Show full item recordAbstract
<jats:title>Abstract</jats:title><jats:p>Estimating the pose of multiple animals is a challenging computer vision problem: frequent interactions cause occlusions and complicate the association of detected keypoints to the correct individuals, as well as having highly similar looking animals that interact more closely than in typical multi-human scenarios. To take up this challenge, we build on DeepLabCut, an open-source pose estimation toolbox, and provide high-performance animal assembly and tracking—features required for multi-animal scenarios. Furthermore, we integrate the ability to predict an animal’s identity to assist tracking (in case of occlusions). We illustrate the power of this framework with four datasets varying in complexity, which we release to serve as a benchmark for future algorithm development.</jats:p>
Date issued
2022Department
Massachusetts Institute of Technology. Department of Brain and Cognitive SciencesJournal
Nature Methods
Publisher
Springer Science and Business Media LLC
Citation
Lauer, Jessy, Zhou, Mu, Ye, Shaokai, Menegas, William, Schneider, Steffen et al. 2022. "Multi-animal pose estimation, identification and tracking with DeepLabCut." Nature Methods, 19 (4).
Version: Final published version