Uncovering material deformations via machine learning combined with four-dimensional scanning transmission electron microscopy
Author(s)
Shi, Chuqiao; Cao, Michael C.; Rehn, Sarah M.; Bae, Sang-Hoon; Kim, Jeehwan; Jones, Matthew R.; Muller, David A.; Han, Yimo; ... Show more Show less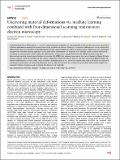
Downloads41524-022-00793-9.pdf (2.195Mb)
Publisher with Creative Commons License
Publisher with Creative Commons License
Creative Commons Attribution
Terms of use
Metadata
Show full item recordAbstract
Understanding lattice deformations is crucial in determining the properties of nanomaterials, which can become more prominent in future applications ranging from energy harvesting to electronic devices. However, it remains challenging to reveal unexpected deformations that crucially affect material properties across a large sample area. Here, we demonstrate a rapid and semi-automated unsupervised machine learning approach to uncover lattice deformations in materials. Our method utilizes divisive hierarchical clustering to automatically unveil multi-scale deformations in the entire sample flake from the diffraction data using four-dimensional scanning transmission electron microscopy (4D-STEM). Our approach overcomes the current barriers of large 4D data analysis without a priori knowledge of the sample. Using this purely data-driven analysis, we have uncovered different types of material deformations, such as strain, lattice distortion, bending contour, etc., which can significantly impact the band structure and subsequent performance of nanomaterials-based devices. We envision that this data-driven procedure will provide insight into materials’ intrinsic structures and accelerate the discovery of materials.
Date issued
2022-05-18Department
Massachusetts Institute of Technology. Department of Mechanical EngineeringJournal
npj Computational Materials
Publisher
Springer Science and Business Media LLC
Citation
Shi, C., Cao, M.C., Rehn, S.M. et al. Uncovering material deformations via machine learning combined with four-dimensional scanning transmission electron microscopy. npj Comput Mater 8, 114 (2022).
Version: Final published version
ISSN
2057-3960
Keywords
Computer Science Applications, Mechanics of Materials, General Materials Science, Modeling and Simulation