Nonlinear wave evolution with data-driven breaking
Author(s)
Eeltink, D.; Branger, H.; Luneau, C.; He, Y.; Chabchoub, A.; Kasparian, J.; van den Bremer, T. S.; Sapsis, T. P.; ... Show more Show less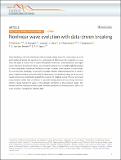
Downloads41467-022-30025-z.pdf (2.192Mb)
Publisher with Creative Commons License
Publisher with Creative Commons License
Creative Commons Attribution
Terms of use
Metadata
Show full item recordAbstract
Wave breaking is the main mechanism that dissipates energy input into ocean waves by wind and transferred across the spectrum by nonlinearity. It determines the properties of a sea state and plays a crucial role in ocean-atmosphere interaction, ocean pollution, and rogue waves. Owing to its turbulent nature, wave breaking remains too computationally demanding to solve using direct numerical simulations except in simple, short-duration circumstances. To overcome this challenge, we present a blended machine learning framework in which a physics-based nonlinear evolution model for deep-water, non-breaking waves and a recurrent neural network are combined to predict the evolution of breaking waves. We use wave tank measurements rather than simulations to provide training data and use a long short-term memory neural network to apply a finite-domain correction to the evolution model. Our blended machine learning framework gives excellent predictions of breaking and its effects on wave evolution, including for external data.
Date issued
2022-04-29Department
Massachusetts Institute of Technology. Department of Mechanical EngineeringJournal
Nature Communications
Publisher
Springer Science and Business Media LLC
Citation
Eeltink, D., Branger, H., Luneau, C. et al. Nonlinear wave evolution with data-driven breaking. Nat Commun 13, 2343 (2022).
Version: Final published version
ISSN
2041-1723
Keywords
General Physics and Astronomy, General Biochemistry, Genetics and Molecular Biology, General Chemistry, Multidisciplinary