Accelerating amorphous polymer electrolyte screening by learning to reduce errors in molecular dynamics simulated properties
Author(s)
Xie, Tian; France-Lanord, Arthur; Wang, Yanming; Lopez, Jeffrey; Stolberg, Michael A.; Hill, Megan; Leverick, Graham Michael; Gomez-Bombarelli, Rafael; Johnson, Jeremiah A.; Shao-Horn, Yang; Grossman, Jeffrey C.; ... Show more Show less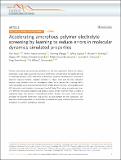
DownloadPublished version (1.323Mb)
Publisher with Creative Commons License
Publisher with Creative Commons License
Creative Commons Attribution
Terms of use
Metadata
Show full item recordAbstract
Polymer electrolytes are promising candidates for the next generation lithium-ion battery technology. Large scale screening of polymer electrolytes is hindered by the significant cost of molecular dynamics (MD) simulation in amorphous systems: the amorphous structure of polymers requires multiple, repeated sampling to reduce noise and the slow relaxation requires long simulation time for convergence. Here, we accelerate the screening with a multi-task graph neural network that learns from a large amount of noisy, unconverged, short MD data and a small number of converged, long MD data. We achieve accurate predictions of 4 different converged properties and screen a space of 6247 polymers that is orders of magnitude larger than previous computational studies. Further, we extract several design principles for polymer electrolytes and provide an open dataset for the community. Our approach could be applicable to a broad class of material discovery problems that involve the simulation of complex, amorphous materials.
Date issued
2022-06-14Department
Massachusetts Institute of Technology. Department of Materials Science and Engineering; Massachusetts Institute of Technology. Computer Science and Artificial Intelligence Laboratory; Massachusetts Institute of Technology. Research Laboratory of Electronics; Massachusetts Institute of Technology. Department of Chemistry; Massachusetts Institute of Technology. Department of Mechanical EngineeringJournal
Nature Communications
Publisher
Springer Science and Business Media LLC
Citation
Xie, T., France-Lanord, A., Wang, Y. et al. Accelerating amorphous polymer electrolyte screening by learning to reduce errors in molecular dynamics simulated properties. Nat Commun 13, 3415 (2022).
Version: Final published version
ISSN
2041-1723