Matching and Predicting Street Level Images
Author(s)
Kaneva, Biliana K.; Sivic, Josef; Torralba, Antonio; Avidan, Shai; Freeman, William T.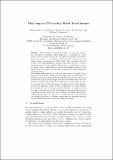
DownloadTorralba_Matching and.pdf (7.720Mb)
OPEN_ACCESS_POLICY
Open Access Policy
Creative Commons Attribution-Noncommercial-Share Alike
Terms of use
Metadata
Show full item recordAbstract
The paradigm of matching images to a very large dataset
has been used for numerous vision tasks and is a powerful one. If the
image dataset is large enough, one can expect to nd good matches of
almost any image to the database, allowing label transfer [3, 15], and
image editing or enhancement [6, 11]. Users of this approach will want
to know how many images are required, and what features to use for
nding semantic relevant matches. Furthermore, for navigation tasks or
to exploit context, users will want to know the predictive quality of the
dataset: can we predict the image that would be seen under changes in
camera position?
We address these questions in detail for one category of images: street
level views. We have a dataset of images taken from an enumeration of
positions and viewpoints within Pittsburgh.We evaluate how well we can
match those images, using images from non-Pittsburgh cities, and how
well we can predict the images that would be seen under changes in cam-
era position. We compare performance for these tasks for eight di erent
feature sets, nding a feature set that outperforms the others (HOG).
A combination of all the features performs better in the prediction task
than any individual feature. We used Amazon Mechanical Turk workers
to rank the matches and predictions of di erent algorithm conditions by
comparing each one to the selection of a random image. This approach
can evaluate the e cacy of di erent feature sets and parameter settings
for the matching paradigm with other image categories.
Date issued
2010-09Department
Massachusetts Institute of Technology. Department of Electrical Engineering and Computer ScienceJournal
Proceedings of the 11th European Conference on Computer Vision, ECCV 2010
Citation
Kaneva, Biliana et al. "Matching and Predicting Street Level Images." in Proceedings of the 11th European Conference on Computer Vision, ECCV 2010, 5-11 Sept. 2010. Hersonissos, Heraklion, Crete, Greece.
Version: Author's final manuscript