A Monte-Carlo AIXI Approximation
Author(s)
Veness, Joel; Ng, Kee Siong; Hutter, Marcus; Uther, William; Silver, David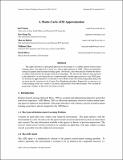
DownloadVeness-2011-A Monte-Carlo AIXI Approximation.pdf (668.2Kb)
PUBLISHER_POLICY
Publisher Policy
Article is made available in accordance with the publisher's policy and may be subject to US copyright law. Please refer to the publisher's site for terms of use.
Terms of use
Metadata
Show full item recordAbstract
This paper introduces a principled approach for the design of a scalable general reinforcement learning agent. Our approach is based on a direct approximation of AIXI, a Bayesian optimality notion for general reinforcement learning agents. Previously, it has been unclear whether the theory of AIXI could motivate the design of practical algorithms. We answer this hitherto open question in the affirmative, by providing the first computationally feasible approximation to the AIXI agent. To develop our approximation, we introduce a new Monte-Carlo Tree Search algorithm along with an agent-specific extension to the Context Tree Weighting algorithm. Empirically, we present a set of encouraging results on a variety of stochastic and partially observable domains. We conclude by proposing a number of directions for future research.
Date issued
2011-01Department
Massachusetts Institute of Technology. Computer Science and Artificial Intelligence LaboratoryJournal
Journal of Artificial Intelligence Research
Publisher
AI Access Foundation
Citation
Veness, Joel et al. (2011) "A Monte-Carlo AIXI Approximation", Journal of Artificial Intelligence Research (2011) Volume 40, pages 95-142. © 2011 AI Access Foundation
Version: Final published version
ISSN
1943-5037
1076-9757