Theory Acquisition as Stochastic Search
Author(s)
Ullman, Tomer David; Goodman, Noah D.; Tenenbaum, Joshua B.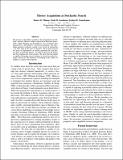
DownloadTenenbaum-2010-Theory Acquisition as Stochastic Search.pdf (1.508Mb)
OPEN_ACCESS_POLICY
Open Access Policy
Creative Commons Attribution-Noncommercial-Share Alike
Terms of use
Metadata
Show full item recordAbstract
We present an algorithmic model for the development of children’s
intuitive theories within a hierarchical Bayesian framework,
where theories are described as sets of logical laws
generated by a probabilistic context-free grammar. Our algorithm
performs stochastic search at two levels of abstraction
– an outer loop in the space of theories, and an inner loop in
the space of explanations or models generated by each theory
given a particular dataset – in order to discover the theory
that best explains the observed data. We show that this model
is capable of learning correct theories in several everyday domains,
and discuss the dynamics of learning in the context of
children’s cognitive development.
Date issued
2010-08Department
Massachusetts Institute of Technology. Department of Brain and Cognitive SciencesJournal
Proceedings of the Thirty-Second Annual Conference of the Cognitive Science Society (CogSci 2010)
Publisher
Cognitive Science Society, Inc.
Citation
Ullman, Tomer D., Noah D. Goodman and Joshua B. Tenenbaum. "Theory Acquisition as Stochastic Search." in Proceedings of the 32nd Annual Meeting of the Cognitive Science Society, COGSCI 2010, Portland, Oregon, August 11-14, 2010.
Version: Author's final manuscript