No Evidence for an Item Limit in Change Detection
Author(s)
Keshvari, Shaiyan Oliver; Berg, Ronald van den; Ma, Wei Ji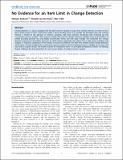
DownloadKeshvari-2013-No Evidence for an I.pdf (736.2Kb)
PUBLISHER_CC
Publisher with Creative Commons License
Creative Commons Attribution
Terms of use
Metadata
Show full item recordAbstract
Change detection is a classic paradigm that has been used for decades to argue that working memory can hold no more than a fixed number of items (“item-limit models”). Recent findings force us to consider the alternative view that working memory is limited by the precision in stimulus encoding, with mean precision decreasing with increasing set size (“continuous-resource models”). Most previous studies that used the change detection paradigm have ignored effects of limited encoding precision by using highly discriminable stimuli and only large changes. We conducted two change detection experiments (orientation and color) in which change magnitudes were drawn from a wide range, including small changes. In a rigorous comparison of five models, we found no evidence of an item limit. Instead, human change detection performance was best explained by a continuous-resource model in which encoding precision is variable across items and trials even at a given set size. This model accounts for comparison errors in a principled, probabilistic manner. Our findings sharply challenge the theoretical basis for most neural studies of working memory capacity.
Date issued
2013-02Department
Massachusetts Institute of Technology. Department of Brain and Cognitive Sciences; Massachusetts Institute of Technology. School of ScienceJournal
PLoS Computational Biology
Publisher
Public Library of Science
Citation
Keshvari, Shaiyan, Ronald van den Berg, and Wei Ji Ma. “No Evidence for an Item Limit in Change Detection.” Ed. Laurence T. Maloney. PLoS Computational Biology 9.2 (2013): e1002927.
Version: Final published version
ISSN
1553-734X
1553-7358