Actor-Critic Policy Learning in Cooperative Planning
Author(s)
Redding, Joshua; Geramifard, Alborz; Choi, Han-Lim; How, Jonathan P.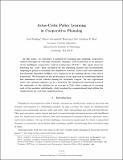
DownloadHow_Actor-critic policy.pdf (489.0Kb)
OPEN_ACCESS_POLICY
Open Access Policy
Creative Commons Attribution-Noncommercial-Share Alike
Terms of use
Metadata
Show full item recordAbstract
In this paper, we introduce a method for learning and adapting cooperative control strategies in real-time stochastic domains. Our framework is an instance of the intelligent cooperative control architecture (iCCA)[superscript 1]. The agent starts by following the "safe" plan calculated by the planning module and incrementally adapting its policy to maximize the cumulative rewards. Actor-critic and consensus-based bundle algorithm (CBBA) were employed as the building blocks of the iCCA framework. We demonstrate the performance of our approach by simulating limited fuel unmanned aerial vehicles aiming for stochastic targets. In one experiment where the optimal solution can be calculated, the integrated framework boosted the optimality of the solution by an average of %10, when compared to running each of the modules individually, while keeping the computational load within the requirements for real-time implementation.
Date issued
2010-08Department
Massachusetts Institute of Technology. Aerospace Controls Laboratory; Massachusetts Institute of Technology. Department of Aeronautics and Astronautics; Massachusetts Institute of Technology. Laboratory for Information and Decision SystemsJournal
Proceedings of the AIAA Guidance, Navigation, and Control Conference
Publisher
American Institute of Aeronautics and Astronautics
Citation
Redding, Joshua, Alborz Geramifard, Han-Lim Choi, and Jonathan How. “Actor-Critic Policy Learning in Cooperative Planning.” In AIAA Guidance, Navigation, and Control Conference. American Institute of Aeronautics and Astronautics, 2010.
Version: Author's final manuscript
ISBN
978-1-60086-962-4
ISSN
1946-9802