Adaptive Planning for Markov Decision Processes with Uncertain Transition Models via Incremental Feature Dependency Discovery
Author(s)
Geramifard, Alborz; Chowdhary, Girish; How, Jonathan P.; Ure, Nazim Kemal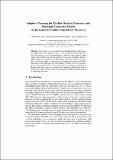
DownloadHow_Adaptive planning.pdf (623.4Kb)
OPEN_ACCESS_POLICY
Open Access Policy
Creative Commons Attribution-Noncommercial-Share Alike
Terms of use
Metadata
Show full item recordAbstract
Solving large scale sequential decision making problems without prior knowledge of the state transition model is a key problem in the planning literature. One approach to tackle this problem is to learn the state transition model online using limited observed measurements. We present an adaptive function approximator (incremental Feature Dependency Discovery (iFDD)) that grows the set of features online to approximately represent the transition model. The approach leverages existing feature-dependencies to build a sparse representation of the state transition model. Theoretical analysis and numerical simulations in domains with state space sizes varying from thousands to millions are used to illustrate the benefit of using iFDD for incrementally building transition models in a planning framework.
Date issued
2012-09Department
Massachusetts Institute of Technology. Department of Aeronautics and Astronautics; Massachusetts Institute of Technology. Laboratory for Information and Decision SystemsJournal
Machine Learning and Knowledge Discovery in Databases
Publisher
Springer-Verlag
Citation
Ure, N.Kemal et al. “Adaptive Planning for Markov Decision Processes with Uncertain Transition Models via Incremental Feature Dependency Discovery.” Machine Learning and Knowledge Discovery in Databases. Ed. PeterA. Flach, Tijl Bie, and Nello Cristianini. Vol. 7524. Springer Berlin Heidelberg, 2012. 99–115. Lecture Notes in Computer Science.
Version: Author's final manuscript
ISBN
978-3-642-33485-6
978-3-642-33486-3
ISSN
0302-9743
1611-3349