Accelerated parallel magnetic resonance imaging reconstruction using joint estimation with a sparse signal model
Author(s)
Weller, Daniel S.; Polimeni, Jonathan R.; Grady, Leo; Wald, Lawrence L.; Adalsteinsson, Elfar; Goyal, Vivek K.; ... Show more Show less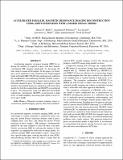
DownloadAdalstein_Accelerated parallel.pdf (419.0Kb)
OPEN_ACCESS_POLICY
Open Access Policy
Creative Commons Attribution-Noncommercial-Share Alike
Terms of use
Metadata
Show full item recordAbstract
Accelerating magnetic resonance imaging (MRI) by reducing the number of acquired k-space scan lines benefits conventional MRI significantly by decreasing the time subjects remain in the magnet. In this paper, we formulate a novel method for Joint estimation from Undersampled LinEs in Parallel MRI (JULEP) that simultaneously calibrates the GeneRalized Autocalibrating Partially Parallel Acquisitions (GRAPPA) reconstruction kernel and reconstructs the full multi-channel k-space. We employ a joint sparsity signal model for the channel images in conjunction with observation models for both the acquired data and GRAPPA reconstructed k-space. We demonstrate using real MRI data that JULEP outperforms conventional GRAPPA reconstruction at high levels of undersampling, increasing the peak-signal-to-noise ratio by up to 10 dB.
Date issued
2012-08Department
Massachusetts Institute of Technology. Department of Electrical Engineering and Computer Science; Massachusetts Institute of Technology. Research Laboratory of ElectronicsJournal
Proceedings of the 2012 IEEE Statistical Signal Processing Workshop (SSP)
Publisher
Institute of Electrical and Electronics Engineers (IEEE)
Citation
Weller, Daniel S., Jonathan R. Polimeni, Leo Grady, Lawrence L. Wald, Elfar Adalsteinsson, and Vivek K Goyal. “Accelerated Parallel Magnetic Resonance Imaging Reconstruction Using Joint Estimation with a Sparse Signal Model.” 2012 IEEE Statistical Signal Processing Workshop (SSP) (n.d.).
Version: Author's final manuscript
ISBN
978-1-4673-0182-4
978-1-4673-0181-7