Learning with Hierarchical-Deep Models
Author(s)
Salakhutdinov, R.; Tenenbaum, Joshua B.; Torralba, Antonio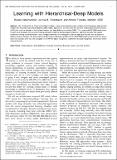
DownloadHD_PAMI.pdf (1.728Mb)
OPEN_ACCESS_POLICY
Open Access Policy
Creative Commons Attribution-Noncommercial-Share Alike
Terms of use
Metadata
Show full item recordAbstract
We introduce HD (or “Hierarchical-Deep”) models, a new compositional learning architecture that integrates deep learning models with structured hierarchical Bayesian (HB) models. Specifically, we show how we can learn a hierarchical Dirichlet process (HDP) prior over the activities of the top-level features in a deep Boltzmann machine (DBM). This compound HDP-DBM model learns to learn novel concepts from very few training example by learning low-level generic features, high-level features that capture correlations among low-level features, and a category hierarchy for sharing priors over the high-level features that are typical of different kinds of concepts. We present efficient learning and inference algorithms for the HDP-DBM model and show that it is able to learn new concepts from very few examples on CIFAR-100 object recognition, handwritten character recognition, and human motion capture datasets.
Date issued
2013-08Department
Massachusetts Institute of Technology. Computer Science and Artificial Intelligence Laboratory; Massachusetts Institute of Technology. Department of Brain and Cognitive Sciences; Massachusetts Institute of Technology. Department of Electrical Engineering and Computer ScienceJournal
IEEE Transactions on Pattern Analysis and Machine Intelligence
Publisher
Institute of Electrical and Electronics Engineers (IEEE)
Citation
Salakhutdinov, R., J. B. Tenenbaum, and A. Torralba. “Learning with Hierarchical-Deep Models.” IEEE Trans. Pattern Anal. Mach. Intell. 35, no. 8 (August 2013): 1958–1971.
Version: Author's final manuscript
ISSN
0162-8828
2160-9292