Approximate Bayesian Image Interpretation using Generative Probabilistic Graphics Programs
Author(s)
Mansinghka, Vikash K.; Kulkarni, Tejas Dattatraya; Perov, Yura N.; Tenenbaum, Joshua B.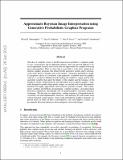
DownloadTenenbaum_Approximate Bayesian.pdf (9.964Mb)
OPEN_ACCESS_POLICY
Open Access Policy
Creative Commons Attribution-Noncommercial-Share Alike
Terms of use
Metadata
Show full item recordAbstract
The idea of computer vision as the Bayesian inverse problem to computer graphics has a long history and an appealing elegance, but it has proved difficult to directly implement. Instead, most vision tasks are approached via complex bottom-up processing pipelines. Here we show that it is possible to write short, simple probabilistic graphics programs that define flexible generative models and to automatically invert them to interpret real-world images. Generative probabilistic graphics programs (GPGP) consist of a stochastic scene generator, a renderer based on graphics software, a stochastic likelihood model linking the renderer’s output and the data, and latent variables that adjust the fidelity of the renderer and the tolerance of the likelihood. Representations and algorithms from computer graphics are used as the deterministic backbone for highly approximate and stochastic generative models. This formulation combines probabilistic programming, computer graphics, and approximate Bayesian computation, and depends only on general-purpose, automatic inference techniques. We describe two applications: reading sequences of degraded and adversarially obscured characters, and inferring 3D road models from vehicle-mounted camera images. Each of the probabilistic graphics programs we present relies on under 20 lines of probabilistic code, and yields accurate, approximately Bayesian inferences about real-world images.
Date issued
2013Department
Massachusetts Institute of Technology. Computer Science and Artificial Intelligence Laboratory; Massachusetts Institute of Technology. Department of Brain and Cognitive SciencesJournal
Advances in neural information processing systems 26, NIPS 2013
Publisher
Neural Information Processing Systems Foundation
Citation
Mansinghka, Vikash K., Tejas D. Kulkarni, Yura N. Perov, and Joshua B. Tenenbaum. Approximate Bayesian Image Interpretation using Generative Probabilistic Graphics Programs." NIPS (2013). p.1520-1528.
Version: Original manuscript
ISSN
1049-5258