Classification without labels: learning from mixed samples in high energy physics
Author(s)
Nachman, Benjamin; Metodiev, Eric Mario; Thaler, Jesse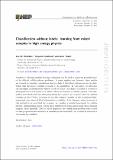
Download13130_2017_Article_6933.pdf (522.9Kb)
PUBLISHER_CC
Publisher with Creative Commons License
Creative Commons Attribution
Terms of use
Metadata
Show full item recordAbstract
Modern machine learning techniques can be used to construct powerful models for difficult collider physics problems. In many applications, however, these models are trained on imperfect simulations due to a lack of truth-level information in the data, which risks the model learning artifacts of the simulation. In this paper, we introduce the paradigm of classification without labels (CWoLa) in which a classifier is trained to distinguish statistical mixtures of classes, which are common in collider physics. Crucially, neither individual labels nor class proportions are required, yet we prove that the optimal classifier in the CWoLa paradigm is also the optimal classifier in the traditional fully-supervised case where all label information is available. After demonstrating the power of this method in an analytical toy example, we consider a realistic benchmark for collider physics: distinguishing quark- versus gluon-initiated jets using mixed quark/gluon training samples. More generally, CWoLa can be applied to any classification problem where labels or class proportions are unknown or simulations are unreliable, but statistical mixtures of the classes are available.
Date issued
2017-10Department
Massachusetts Institute of Technology. Center for Theoretical Physics; Massachusetts Institute of Technology. Department of PhysicsJournal
Journal of High Energy Physics
Publisher
Springer Berlin Heidelberg
Citation
Metodiev, Eric M., et al. “Classification without Labels: Learning from Mixed Samples in High Energy Physics.” Journal of High Energy Physics, vol. 2017, no. 10, Oct. 2017.
Version: Final published version
ISSN
1029-8479
1126-6708