Generative Oversampling with a Contrastive Variational Autoencoder
Author(s)
Dai, Wangzhi; Ng, Kenney; Severson, Kristen A; Huang, Wei; Anderson, Fred; Stultz, Collin M; ... Show more Show less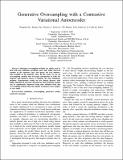
DownloadAccepted version (1.879Mb)
Open Access Policy
Open Access Policy
Creative Commons Attribution-Noncommercial-Share Alike
Terms of use
Metadata
Show full item recordAbstract
© 2019 IEEE. Although oversampling methods are widely used to deal with class imbalance problems, most only utilize observed samples in the minority class and ignore the rich information available in the majority class. In this work, we use an oversampling method that leverages information in both the majority and minority classes to mitigate the class imbalance problem. Experimental results on two clinical datasets with highly imbalanced outcomes demonstrate that prediction models can be significantly improved using data obtained from this oversampling method when the number of minority class samples is very small.
Date issued
2019-11Department
Massachusetts Institute of Technology. Department of Electrical Engineering and Computer Science; Massachusetts Institute of Technology. Computer Science and Artificial Intelligence Laboratory; MIT-IBM Watson AI Lab; Massachusetts Institute of Technology. Institute for Medical Engineering & ScienceJournal
Proceedings - IEEE International Conference on Data Mining, ICDM
Publisher
Institute of Electrical and Electronics Engineers (IEEE)
Citation
Dai, Wangzhi, Ng, Kenney, Severson, Kristen, Huang, Wei, Anderson, Fred et al. 2019. "Generative Oversampling with a Contrastive Variational Autoencoder." Proceedings - IEEE International Conference on Data Mining, ICDM, 2019-November.
Version: Author's final manuscript